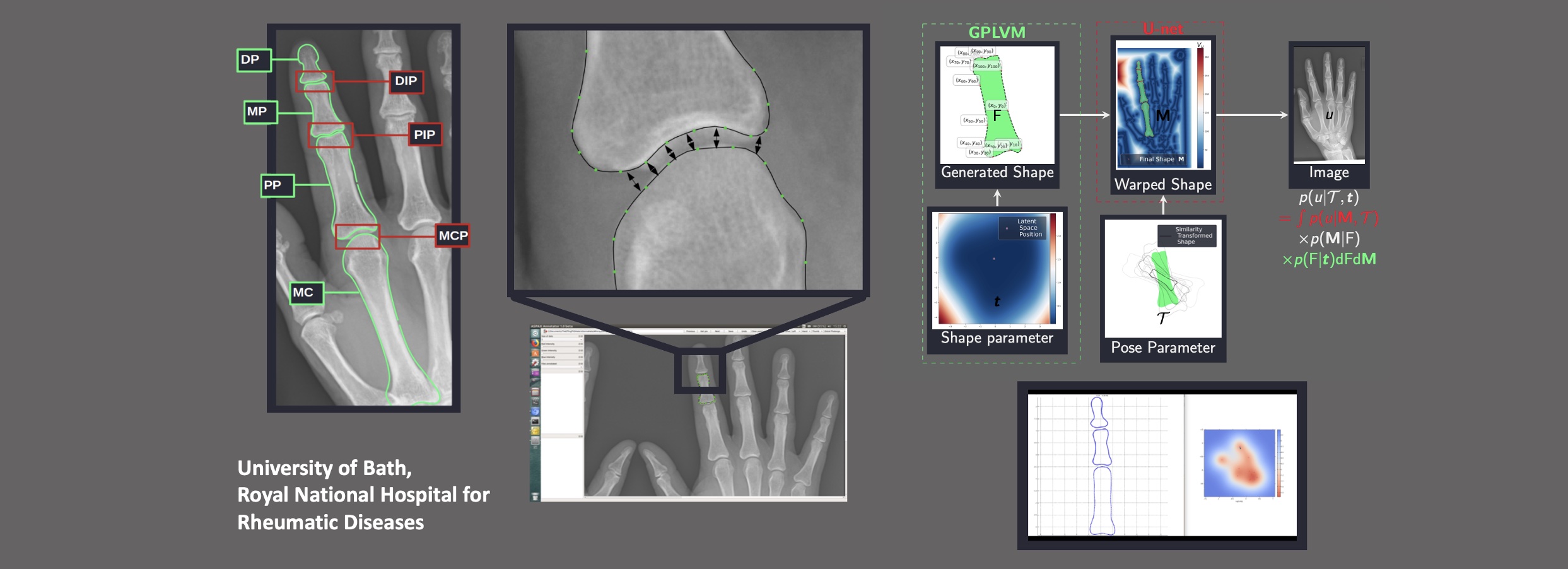
PhD Position: Machine Learning for Structural Damage Detection in Psoriatic Arthritis Hand Radiographs
There is an exciting opportunity to develop new generative machine learning models to help with the diagnosis and treatment of arthritis (a sadly common disease affecting one in five people living with psoriasis and more than >120,000 sufferers in uk alone).
Existing collaborative work with the UK national centre for arthritis has made a large amount of data available in the form of hand x-rays and the project is sponsored by Pfizer who are very interested in the results to develop and validate better drugs with the long-term aim of halting the progression on the disease before too much damage is done.
On a technical front we will develop new state-of-the-art generative machine learning models and combine them with work from medical imaging (e.g. inverse problems) to produce accurate 3D models of the hand from cheap and accessible 2D x-rays and infer clinically relevant information (e.g. disease progression) to help identify the optimal treatments.
You will be working within a team of people across computer science, mathematical and clinical expertise and your work will create real impact in the field with the resulting software being put to use directly by clinicians and scientists at the top of the field. Your results will be published at the top conferences and journals and the project will provide excellent training for a research career in academia or industry afterwards.
Further background information:
Plain radiography and the quantification of radiographic damage is an important outcome in Psoriatic Arthritis (PsA) from the patient and clinician perspective. Detailed scoring methods for more accurate assessment of radiographic damage are available but very time consuming so rarely performed. An automated process with greater accuracy would have the potential of increasing the accuracy of damage detection in clinical trials as well as large observational cohorts to answer important questions on diagnosis and response to treatment.